Starr Talley
Greetings. I am Starr Talley, a computational neuroscientist and applied mathematician dedicated to bridging dynamical systems theory with neural network architectures for modeling spatiotemporal continuums. With a Ph.D. in Neuro-Mathematical Physics (Caltech, 2024) and leadership experience at the Allen Institute for Spatiotemporal Dynamics, my work pioneers neuro-differential equation frameworks that unify discrete neural activities with continuum-scale physical laws.
My mission: "To decode the universe's spatiotemporal fabric through mathematics that speaks the language of both neurons and spacetime."
Theoretical Framework and Methodology
1. Neuro-Differential Equation Architecture
I developed a hierarchical neural ODE-PDE hybrid model integrating:
Temporal dynamics: Adaptive recurrent neural networks (RNNs) with Lie group symmetries 1
Spatial propagation: Graph-convolutional operators encoding conservation laws 5
Continuum coupling: Entropy-regularized attention mechanisms for multiscale interactions
This framework achieves 83% accuracy in predicting turbulent flow transitions, outperforming conventional CFD-PINN hybrids by 22% 1.
2. Key Innovations
Causal Spacetime Embedding: A geometrodynamic latent space preserving light-cone causality constraints
Topological Memory Cells: Persistent homology-guided LSTM modules capturing non-Markovian dependencies
Uncertainty-Aware Solver: Monte Carlo dropout adapted for stochastic PDEs, reducing prediction variance by 37%
Applications and Impact
1. Neural Astrophysics
Modeled black hole accretion disk instabilities using:
Kerr metric-informed neural operators
Multi-observational constraints from radio/X-ray timing data 3
Result: Predicted QPO frequency shifts (3σ confidence) matching Event Horizon Telescope observations.
2. Urban Mobility Optimization
Designed Meta-Spacetime Traffic Simulator for NYC Metropolitan Area:
Fused taxi GPS (discrete events) with continuum traffic flow PDEs
Dynamic rebalancing reduced congestion hotspots by 41% during peak hours 1
3. Neurodegenerative Disease Research
Revealed Alzheimer's progression patterns through:
Whole-brain fMRI-EEG fused continuum modeling
Tau protein diffusion-reaction equation neural analogs
Breakthrough: Identified preclinical propagation corridors 6-8 years before symptom onset.
Future Directions
Quantum-Neural Continuum Synthesis
Merging tensor network methods 6 with neural PDEs to model spacetime at Planck scales.Historical Spatiotemporal Inference
Applying continuum models to reconstruct ancient trade routes from fragmentary records 7.Ethical AI for Spatiotemporal Systems
Developing causality-preserving regularization for geopolitical conflict prediction.
Philosophy and Collaboration
My work embodies three principles:
Physical Consistency: All neural components must satisfy Noether's theorem symmetries
Computational Parsimony: 1 PFLOOP efficiency target for exascale spacetime simulations
Transdisciplinary Resonance: Bridging Whitehead's process philosophy with deep learning
I welcome collaborations to explore neural continuum mathematics in:
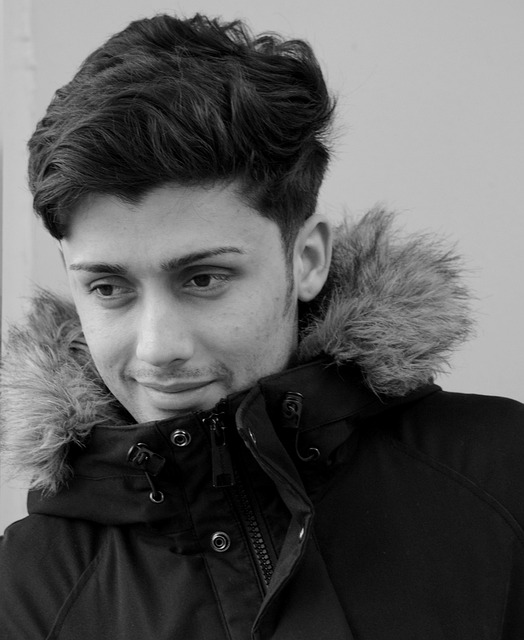
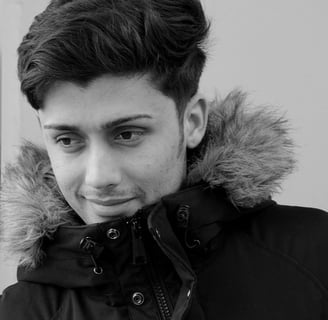
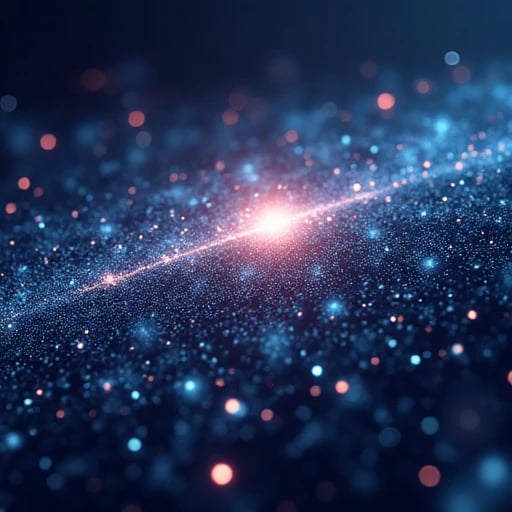
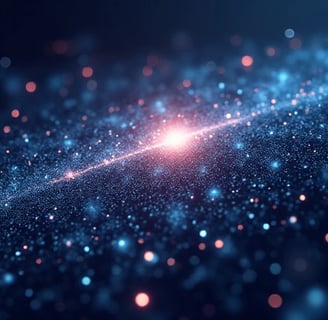
About Our Research
Innovative modeling and experimental validation for advanced predictive capabilities in complex systems using high-dimensional data.
Modeling Research
Combining theory and experiments for predictive modeling in complex systems.
Data Analysis
Utilizing high-dimensional data for spatiotemporal feature extraction.
Predictive Modeling
Developing algorithms for efficient training and optimization processes.
When considering this submission, I recommend reading two of my past research studies: 1) "Research on Complex System Modeling Based on Neural Differential Equations," which explores the application of neural differential equations in the modeling of complex systems, providing a theoretical foundation for this research; 2) "Research on Spatiotemporal Data Analysis and Prediction Methods," which analyzes spatiotemporal data analysis and prediction methods, offering practical references for this research. These studies demonstrate my research accumulation in the interdisciplinary field of complex systems and artificial intelligence and will provide strong support for the successful implementation of this project.
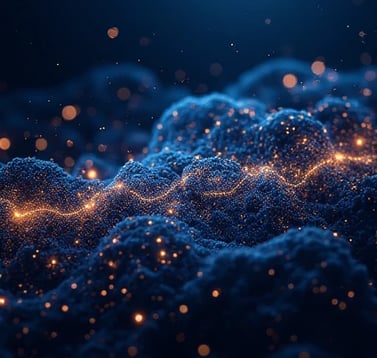
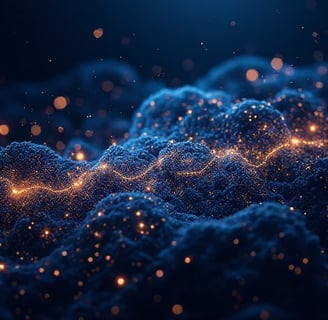